In many organizations, the design team does some research then retreats to their tower to conjure deep magics that turn note filled notebooks into a customer journey map. At least that’s what it looks like to their peers. Journey diagrams capture tons of detailed info about users, processes, and systems. The best teams share the same understanding of the user’s journey. Instead of having your team wonder where you got this information or how you came to these conclusions, have
Continue reading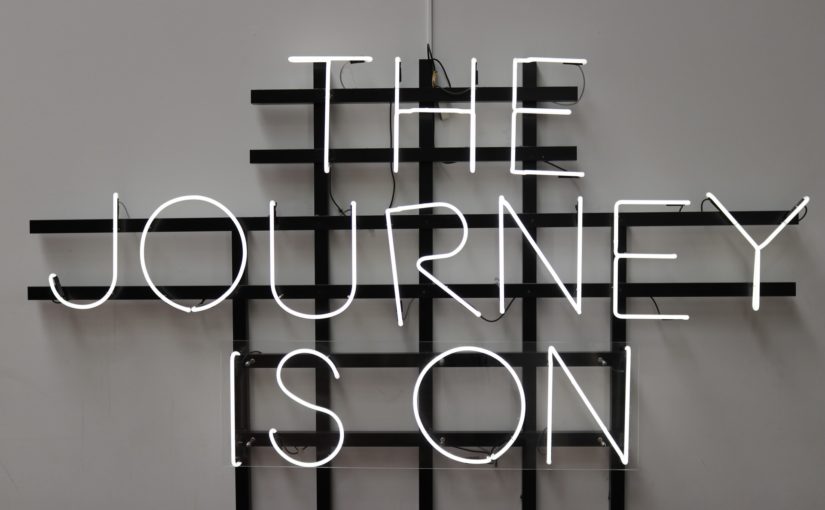